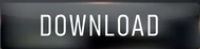
Important connections between genes are detectable only if we consider the data as a whole: each row representing the many measurements made on the same observational unit. We would miss a lot of important information if we were to only study each gene separately.
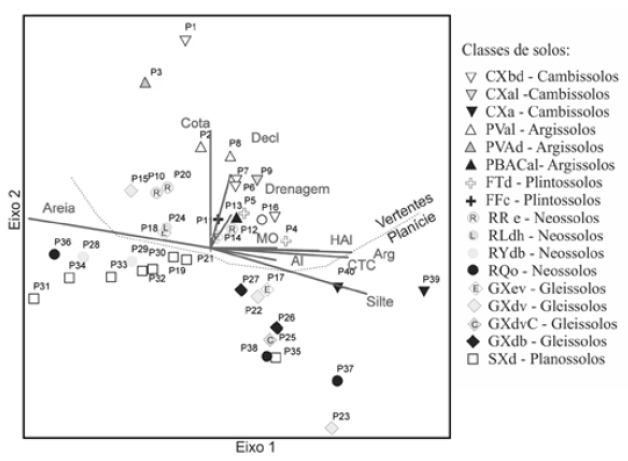
Studying the expression of 25,000 gene (columns) on many samples (rows) of patient-derived cells, we notice that many of the genes act together, either that they are positively correlated or that they are anti-correlated. For instance, in the biology of cells, we know that the proliferation rate will influence the expression of many genes simultaneously. More often, there will be patterns and dependencies. If the columns of the matrix are all independent of each other (unrelated), we can simply study each column separately and do standard “univariate” statistics on them one by one there would be no benefit in studying them as a matrix. In the following, we will focus on the special case where each of the variables is numeric, so we can represent the data structure as a matrix in R. Usually the data are reported in a tabular data structure with one row for each subject and one column for each variable.
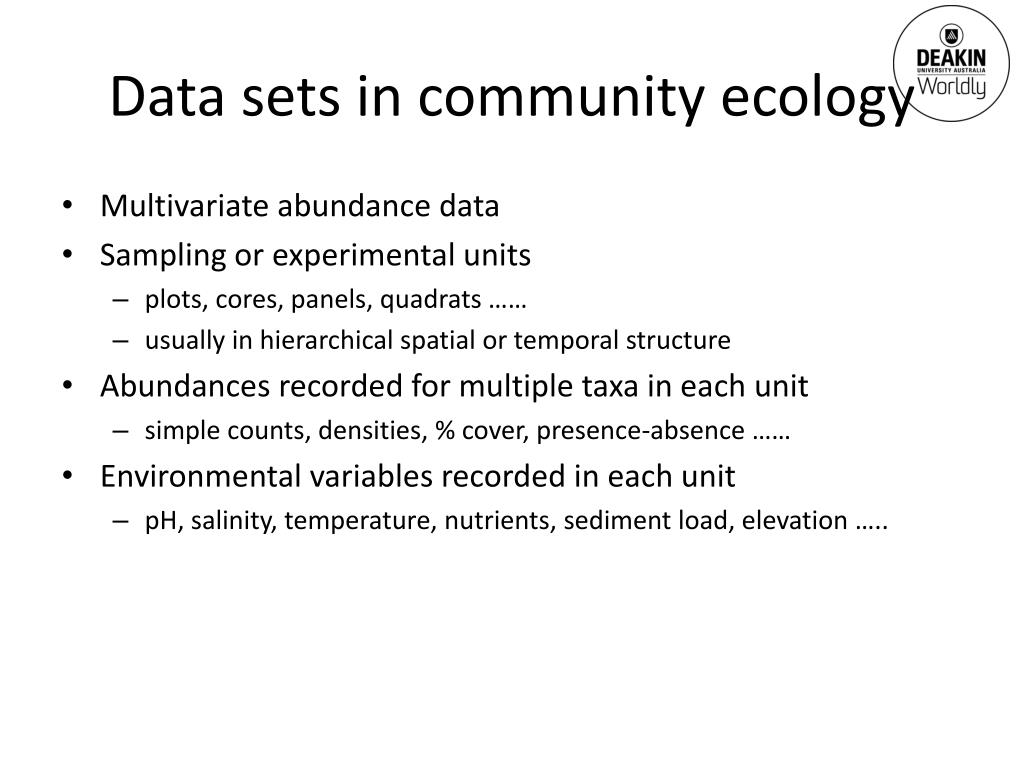

The raison d’être for multivariate analysis is the investigation of connections or associations between the different variables measured. For instance, we may have biometric characteristics such as height, weight, age as well as clinical variables such as blood pressure, blood sugar, heart rate, and genetic data for, say, a thousand patients. Many datasets consist of several variables measured on the same set of subjects: patients, samples, or organisms.
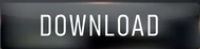